Semi-Structured RAG in Legal Workflows
What is Semi-Structured RAG and Why Does It Matter?
Retrieval Augmented Generation (RAG) has been a groundbreaking approach in the realm of large language models, enabling the generation of responses based on large-scale document databases. However, traditional RAG solutions were primarily designed to handle text-based data, leaving a gap when it comes to documents containing a mix of content types, like text and tables.
Semi-structured data presents distinct challenges for conventional Retrieval Augmented Generation (RAG). First, the process of text splitting might disrupt tables, leading to data corruption during retrieval. Second, embedding these tables can make it difficult to effectively search for semantic similarities.
Enter Semi-Structured RAG, which can parse, summarize, and index both the text and tables from a document, enabling efficient and effective retrieval of information from mixed content types. This ability to leverage information from both text and structured data like tables broadens its applications, particularly in the legal landscape.
Semi-Structured RAG for Legal Research
Attorneys often work with complex documents filled with a mix of text and structured data like tables and lists. These documents can include case files, contracts, legal briefs, and statutes, among others. Processing these documents manually for information retrieval can be time-consuming and prone to errors.
Here's where Semi-Structured RAG comes into play. By automating the extraction and summarization of information from both text and tables, this method streamlines legal research, enhances document analysis, and ultimately saves valuable time for legal professionals. For instance, an attorney could use Semi-Structured RAG to quickly retrieve relevant case law and precedents from a large legal database, thereby accelerating their case preparation process.
How Does Semi-Structured RAG Work?
At its core, Semi-Structured RAG involves three key steps: partitioning, summarization, and retrieval.

- Partitioning/parsing: The process first partitions a document into sections of text and tables. This is like separating the document into different types of content for easier processing. We can seamlessly leverage ETL tools like Unstructured for this step.
- Summarization: Next, we use large language models, like OpenAI’s GPT-4, to generate summaries of these text chunks and tables. These summaries serve as concise representations of the document's content.
- Retrieval and generation: Finally, these summaries are indexed in a retriever. When a query is made, the retriever scans these indexed summaries for relevant information, providing a more comprehensive understanding of the context. LangChain offers an invaluable multi-vector retriever that facilitates this step.
Think of it as having an intelligent assistant who can read through your entire legal database, understand the content, and provide you with a concise summary of the most relevant information in response to your queries.
Ultimately, Semi-Structured RAG represents a significant advancement in legal tech. By harnessing this technology, legal professionals can enhance their document analysis capabilities, streamline their workflows, and ultimately deliver better legal outcomes.
Looking Ahead
As we navigate the fast-evolving landscape of legal tech, Semi-Structured RAG emerges as a powerful tool in our expansive AI toolbox. Its ability to efficiently parse and process both text and structured data from complex documents represents a major leap in our approach to information extraction and summarization. But it's important to remember that while Semi-Structured RAG is transformative, it's just one of the many advanced methods we're integrating.
Harnessing Semi-Structured RAG means empowering attorneys with timely and accurate information extraction, enabling them to focus more on strategic decision-making and client engagement. It brings us a step closer to a future where technology and human expertise work hand in hand, driving better legal outcomes.
As we continue to build and leverage AI tools at Khawaja Partners, Semi-Structured RAG undoubtedly plays a crucial role, especially in enhancing our recruiting services. It aids in delivering precise candidate-law firm matches, reducing placement time, and providing comprehensive candidate profiles, leading to more successful and satisfying legal placement outcomes. Yet, it's a piece of a much larger, holistic strategy aimed at revolutionizing the way we serve our clients and candidates.
Stay Informed
of exclusive market insights*
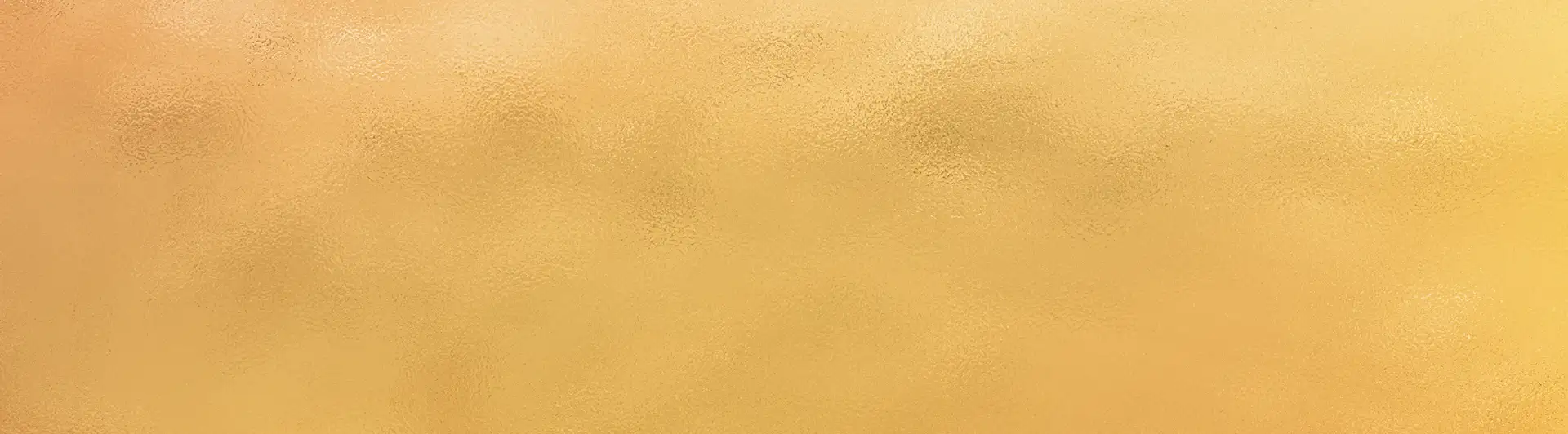
